Regarding these 2 images.

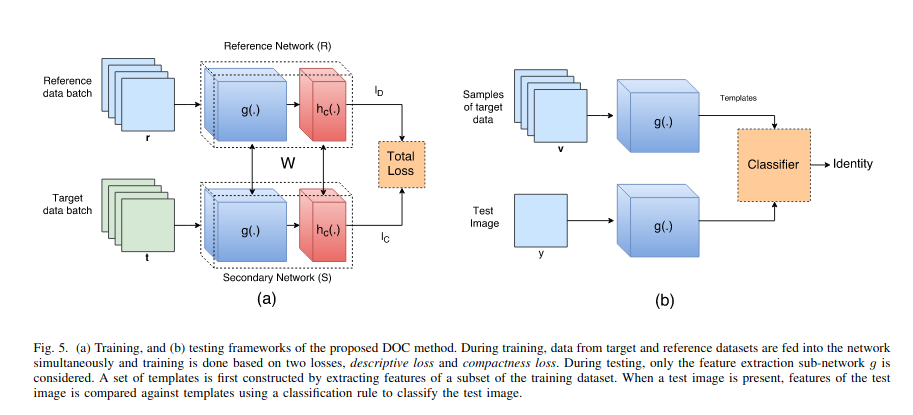
My understanding is that compactness loss should only be calculated from the target batch. And descriptiveness loss should only be calculated from the reference batch.
Am I correct to understand that the weights reference network and secondary network are identical across all gradient updates?
In other words, for the W
in the equation, does that refer to the weights representing the combined weights from the reference network and secondary network, or does that mean the reference network's weights and secondary network's weights are exactly the same set of weights?
If is is the former, then it fine to have 2 network stacks that are being trained from the different loss functions, and their weights will deviate, with the secondary network learning the compactness of the batch, while the reference network being trained to do multi-class classification.
If it is the latter, then I am confused as to how the weights are made identical without freezing entirely both networks.